Fast and scalable protein stability prediction improvement without additional training or data
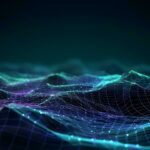
Summary
Deep-learning protein sequence models have shown outstanding performance at de novo protein design and variant effect prediction.
By introducing a second term derived from the models themselves, we substantially improved performance without further training or additional experimental data. This term aligns outputs for the task of stability prediction.
On a task to predict variants that increase protein stability, the absolute success probabilities of ProteinMPNN and ESMif are improved by 11% and 5%, respectively.
Key findings
We demonstrate that inverse folding models can be significantly improved at protein stability prediction without additional training or data.
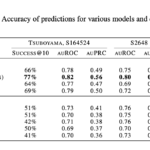
The source of improvement
The improvement in accuracy is achieved through a simple additional term derived from the model itself, where only backbone atoms of the single residue being predicted are given, without sequence or structural context, analogously to standard procedures in classical free energy calculations.
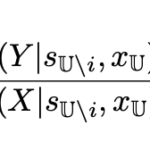
Predicting thousands of residues per second
ProteinMPNN is modified to use full sequence context, and we introduce a novel tied decoding scheme to improve computational efficiency and enable saturation mutagenesis studies at scale.
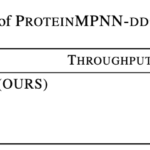
Citing this work
Source code
Interactive Code on Google Colab
https://colab.research.google.com/github/PeptoneLtd/proteinmpnn_ddg/blob/main/ProteinMPNN_ddG.ipynb
About Peptone
Peptone is a translational biophysics company focused on the discovery of novel therapeutics against diseases driven by intrinsically disordered proteins. IDPs are proteins without a fixed structure that play a significant role in health and disease.